Replacing qualitative researchers with AI, a good decision?
Practical examples of AI versus the human researcher
- Article
- Research
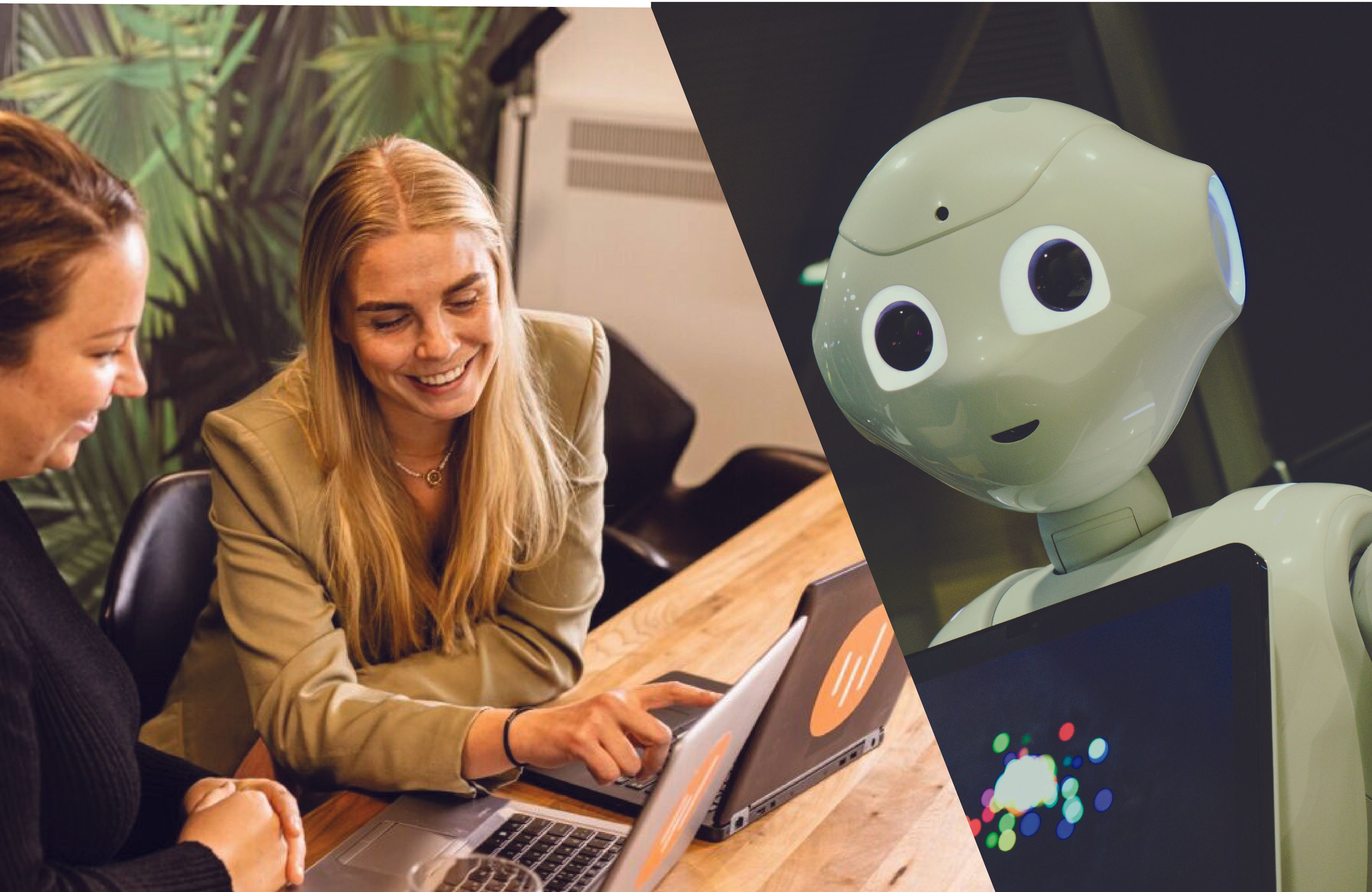
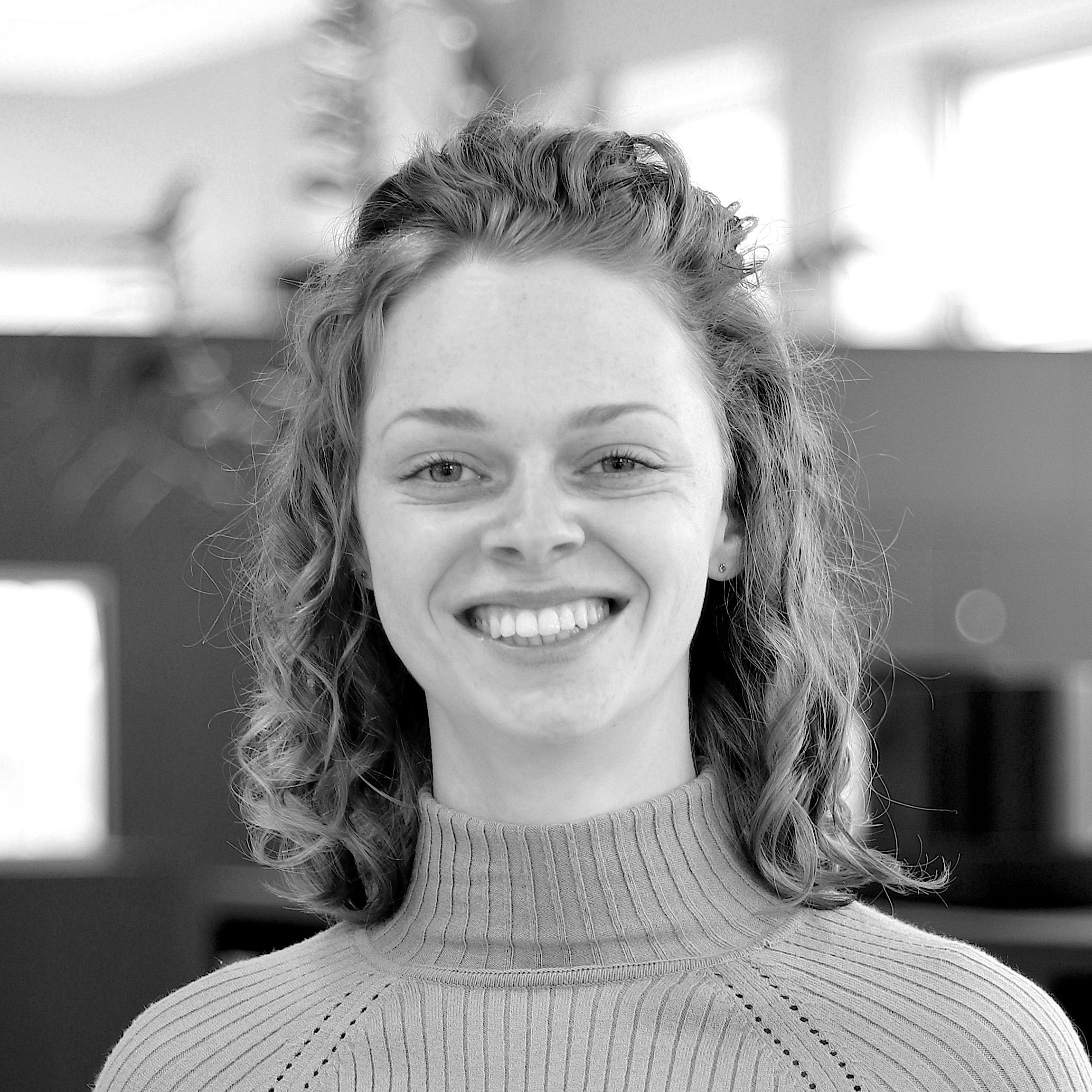
Artificial Intelligence seems capable of everything, and sometimes even better and faster than what we can do ourselves. Analysing qualitative data is a time-consuming task, and as researchers, we are curious if it can be done faster and easier. Does AI offer a solution for this? Our researchers investigated.
Approach
Artificial Intelligence is everywhere in the news and is being increasingly deployed, including for the analysis of qualitative data. Naturally, you don't want the use of AI to lead to a lowering of your quality standard. We investigated the quality of qualitative analyses done with AI tools for you.
For this research, we used various AI tools (ChatGPT 4.0, Survalyzer, Atlas.ti) and our own unsupervised clustering model (Python) to analyze open-ended responses from questionnaires. These responses had already been manually analysed by us. In this article, we present the results of our test: AI versus Digital Power researcher.
AI vs. Researcher: Findings
Black box & fabricated results
Many AI tools are 'black boxes': it is very difficult to understand how they arrive at their results. For scientific research, it is crucial that analyses can be replicated, which is not possible with the black box. Additionally, due to this 'black box', it is often barely possible to check the analysis of the AI tool: which data is categorised in what way?
An example of this occurred during our attempt to analyse qualitative data with ChatGPT 4.0. When we asked for a list of the main findings from the data, we also received 'findings' that did not appear in the data at all, but seemed realistic. We noticed this only because we were already well aware of the content of the data through our 'human' analysis. This is one of the main pitfalls of ChatGPT: it confidently presents information that may be incorrect.
Categorisation into custom categories
In Survalyzer (a tool that uses ChatGPT), we added our own categories. We used the tool to categorise the open-ended responses. But only a fraction (around 10 percent) of the responses were assigned to a category. We also observed a lot of inconsistency in this categorisation: for example, the response 'high travel costs' was not added to the category 'travel', but the response 'travel costs' was.
Another stumbling block was that AI-generated results were often placed in the 'other' category. This resulted in valuable insights being lost. Our manual categorisation proved to be more effective in many cases, with a greater proportion of data being categorised into a relevant theme.
Categorisation into AI-generated categories
AI tools can also be used to generate (initial) categories, into which the data is then categorised. Sometimes, the tools created an excessive number of categories, which were too detailed. For example, in AtlasTi, more than a hundred categories were created for the analysis of a single open question. This makes it difficult to obtain meaningful, overarching insights with categories that are representative of a larger portion of the data.
For the unsupervised clustering model, writing code for this analysis takes a lot of time, just like manual analysis. Additionally, the model needs sufficient information (i.e., responses in open questions) to be able to say something useful.
AI: useful for qualitative analysis, or not?
The use of AI tools for analysing open survey questions currently adds little value. At the moment, AI is not advanced enough for you to simply throw your data into a tool and get reliable results. Of course, efforts are being made to improve these tools, but until then, a lot of manual work will still be necessary. This certainly does not mean that we do not see any possibilities for the use of AI for qualitative analyses.
When could AI be useful?
- If there are already existing, clear categories that closely match the data: a demo of Survalyzer shows promising results in this regard, although this was not the case with our data. You will need to manually create the categories based on a substantial subset of the data first. This will be an iterative process where you need to check how usable the categories are for the AI tool.
- With very large datasets: the larger the dataset, the more data can be used for training. Also, the preparation, as described above, will be especially worthwhile for larger datasets.
- As an additional check on manual analyses, where you test your own results for bias: in the scientific world, controls by peers are often used, such as peer reviews of articles, reproducing research, or calculating inter-rater reliability (the degree of agreement among multiple independent raters performing the same analysis). In practice, it often takes too much time to have these controls performed by colleague researchers. An additional check, for example, by an AI tool, can then be helpful. In the absence of availability of fellow researchers, missed insights and bias can be checked in this way.
Conclusion: the human researcher wins (for now) against AI
At the moment, we have encountered too many problems and limitations to rely on AI tools for qualitative analysis. Also in the near future, we recommend preferring human researchers over AI functionalities for research where deep knowledge of the data is required.
However, at Digital Power, we will continue to monitor developments in the AI world and remain open to new possibilities. We are convinced that AI can play a valuable role in the future of research, but it is essential that people understand the limits and limitations of AI and critically evaluate them.
Finally, what does ChatGPT itself think?
"ChatGPT and similar AI models can be useful in analysing qualitative data from open survey questions, but there are limitations. They need to be trained and validated, and can be biased. Human expertise remains important for deep insights and correcting errors. AI can be especially useful in large datasets to discover patterns but should be supplemented with human analysis for accuracy and context."
We can agree with this.
*The assistance of ChatGPT was enlisted to write this article, but unfortunately did not meet our expectations.
This is an article by Marit
Marit is a researcher at Digital Power. She is enthusiastic about using qualitative and quantitative research methods to understand human behavior, thoughts, and needs. With her background in Human-Technology Interaction, she combines her knowledge of psychology, research, and data analysis to gain insights and develop solutions that contribute to a better user experience. Our Team Lead Research Mieke Kleppe co-wrote this article.
Receive data insights, use cases and behind-the-scenes peeks once a month?
Sign up for our email list and stay 'up to data':